For systems that return a ranked sequence of documents, it is desirable to also consider the order in which the returned documents are presented. Unlike MRR, it considers all the relevant items. My doubt is: if AP changes according to how many objects we retrieve then we can tune this parameter to our advantage so we show the best AP value possible. How do I retrieve decimals when rounding.

In pattern recognition, information retrieval and classification (machine learning), precision (also called positive predictive value) is the fraction of relevant instances among the retrieved instances, while recall (also known as sensitivity) is the fraction of the total amount of relevant instances that were actually retrieved. However, it is quite tricky to interpret and compare the scores that are seen. In this question I asked clarifications about the precision -recall curve.
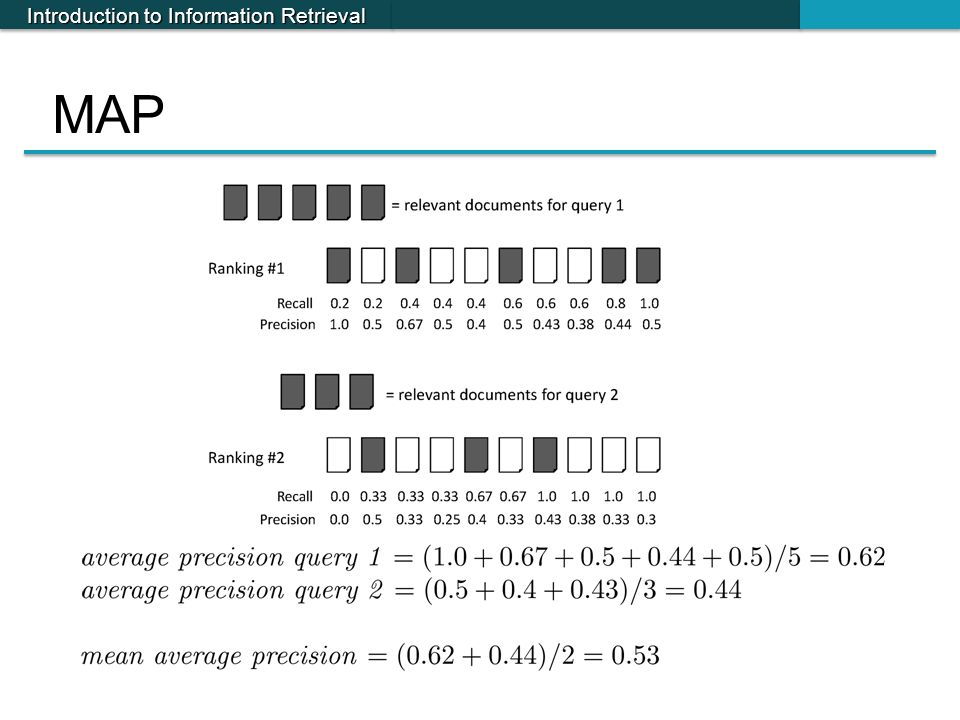
In particular, I asked if we have to consider a fixed number of rankings to draw the curve or we can reasonably choose our. Recall is defined as ratio of the number of retrieved and relevant documents (the number of items retrieved that are relevant to the user and match his needs) to the number of possible relevant documents (number of relevant documents in the database).
The TAP-ksummarizes features of the precision–recall (PR) curve (described in Section 2). Mean average precision (MAP) considers whether all of the relevant items tend to get ranked highly. Average precision (AP) is the average of (un-interpolated) precision values at all ranks where.
Recent deep models for image retrieval have outperformed traditional methods by leveraging ranking-tailored loss functions, but important theoretical and practical problems remain. First, rather than directly optimizing the global ranking, they minimize an upper-bound on the essential loss, which does not necessarily result in an optimal mean average precision (mAP).
Secon these methods. Both these domains have different ways of calculating mAP. We will talk of the Object Detection relevant mAP. Recall, sometimes referred to as ‘sensitivity, is the fraction of retrieved instances among all relevant instances.
Furthermore, in addition to conditions previously given in the literature, we introduce three new criteria that an ideal measure of retrieval efficacy should satisfy. In information retrieval, a perfect precision score of 1. In practice, a higher mAP value indicates a better performance of your neural net, given your ground-truth and set of classes. Precision and Recall. Information retrieval (IR).
When a user decides to search for information on a topic, the total database and the resu. Ask Question Asked years, months ago. Active years, months ago. Jerome Revau et al.
Image retrieval can be formulated as a ranking problem where the goal is to order database images by decreasing similarity to the query. Commonly 10-points of recall is used!
Some measures are more widely used for comparing systems and can be used in order to rank systems. Some methods are tuned in order to enhance a retrieval property, such as high precision for example. VLF-heslap VLF-harlap VLF-SIFT mAP 0. Note, that these values are computed only over small part of the Oxford Buildings dataset, and therefore are not directly comparable to the other state-of-the-art image retrieval systems run on the Oxford Buildings dataset.
Recent research has suggested an alternative, evaluating information retrieval systems based on user behavior. Particularly promising are experiments that interleave two. Virtually all modern evaluation metrics (e.g., mean average precision, discounted cumulative gain) are designed for ranked retrieval without any explicit rank cutoff, taking into account the relative order of the documents retrieved by the search engines and giving more weight to documents returned at higher ranks.
INTRODUCTION The purpose of IR evaluation is to measure the effective-ness, or relative effectiveness, of information retrieval sys-tems. Our objective is to obtain a relative picture of a better IR model for Gujarati Language. That is, if the set of relevant documents for an in- Using MAP, fixed recall levels are not chosen, and there is no interpolation. Traditional measures of retrieval effectiveness such as precision and recall assume the relevance judgments are complete.
However, modern document sets are too large to have a human look at every document for every topic. TREC pioneered the use of pooling to create a smaller subset of documents to judge for a topic. The main assumption underlying pooling is that judging only the top-ranked. Graph comparing the harmonic mean to other means.
The graph shows a slice through the calculation of various means of precision and recall for the fixed recall value of 70%. The harmonic mean is always less than either the arithmetic or geometric mean, and often quite close to the minimum of the two numbers.

When the precision is also 70%, all the measures coincide.
Aucun commentaire:
Enregistrer un commentaire
Remarque : Seul un membre de ce blog est autorisé à enregistrer un commentaire.